基于机器学习和深度学习的蛋白质结构预测研究进展
DOI:
https://doi.org/10.52810/FAAI.2024.003关键词:
蛋白质结构预测, 深度学习, 机器学习, 卷积神经网络, Transformer 模型, 生成式对抗网络摘要
蛋白质结构预测是生物信息学领域的一个核心问题,对于理解蛋白质功能、药物设计以及疾病研究具有重要意义。传统的蛋白质结构预测方法受限于计算复杂度和预测精度。近年来,随着机器学习和深度学习技术的快速发展,这些先进的方法被广泛应用于蛋白质结构预测中,显著提高了预测的准确性和效率。本文首先介绍了蛋白质结构预测的背景和重要性,然后详细阐述了机器学习和深度学习在蛋白质结构预测中的应用,包括常用的算法、模型架构以及优化策略。最后,本文展望了基于机器学习和深度学习的蛋白质结构预测在未来的发展方向和潜在挑战,为相关领域的研究者提供了有价值的参考。
参考文献
Prediction Center. (n.d.). CASP: Critical Assessment of protein Structure Prediction. Retrieved from https://predictioncenter.org/ on March 15, 2023
王栋,孙济洲,李福超,等.基于并行多类支持向量机的蛋白质结构预测[J].计算机应用研究,2011,28(02):465-468.
王菲露,宋杰,宋杨.BP神经网络在蛋白质二级结构预测中的应用[J].计算机技术与发展,2009,19(05):217-219+223.
王菲露,宋杨.基于广义回归神经网络的蛋白质二级结构预测[J].计算机仿真,2012,29(02):184-187.
张斌,尹京苑,薛丹.基于 RBF 神经网络的蛋白质二级结构预测[J].生物信息学,2011,9(03):224-228+234.
WANG S, SUN S Q, LI Z, et al. Accurate De Novo Prediction of Protein Contact Map by Ultra-Deep Learning Model [J]. Plos Computational Biology, 2017, 13(1).
XU J B. Distance-based protein folding powered by deep learning [J]. Proceedings of the National Academy of Sciences of the United States of America, 2019, 116(34): 16856-65.
WU Q, PENG Z L, ANISHCHENKO I, et al. Protein contact prediction using metagenome sequence data and residual neural networks [J]. Bioinformatics, 2020, 36(1): 41-8.
FUKUDA H, TOMII K. DeepECA: an end-to-end learning framework for protein contact prediction from a multiple sequence alignment [J]. Bmc Bioinformatics, 2020, 21(1).
LI Y, ZHANG C X, BELL E W, et al. Deducing high-accuracy protein contact-maps from a triplet of coevolutionary matrices through deep residual convolutional networks [J]. Plos Computational Biology, 2021, 17(3).
JAIN A, TERASHI G, KAGAYA Y, et al. AttentiveDist: Protein Inter-Residue Distance Prediction Using Deep Learning with Attention on Quadruple Multiple Sequence Alignments [J]. bioRxiv, 2020.
JUMPER J, EVANS R, PRITZEL A, et al. Highly accurate protein structure prediction with AlphaFold [J]. Nature, 2021, 596(7873): 583-+.
张弘,王慧洁,鲁睿捷,等.蛋白质结构预测模型AlphaFold2的应用进展[J/OL].生物工程学报:1-14[2024-04-22].https://doi.org/10.13345/j.cjb.230677.
BAEK M, DIMAIO F, ANISHCHENKO I, et al. Accurate prediction of protein structures and interactions using a three-track neural network [J]. Science, 2021, 373(6557): 871-+.
MIRDITA M, SCHüTZE K, MORIWAKI Y, et al. ColabFold: making protein folding accessible to all [J]. Nature Methods, 2022, 19(6): 679-+.
Meng, Q. Z., et al. (2023). "Improved structure-related prediction for insufficient homologous proteins using MSA enhancement and pre-trained language model." Briefings in Bioinformatics 24(4).
LIU S, WU K, CHEN C. Obtaining protein foldability information from computational models of AlphaFold2 and RoseTTAFold [J]. Computational and Structural Biotechnology Journal, 2022, 20: 4481-9.
NGUYEN P T, HARRIS B J, MATEOS D L, et al. Structural modeling of ion channels using AlphaFold2, RoseTTAFold2, and ESMFold [J]. Channels, 2024, 18(1).
WANG W, PENG Z, YANG J. Single-sequence protein structure prediction using supervised transformer protein language models [J]. Nature computational science, 2022, 2(12): 804-14.
杨璐,董洪伟.基于自注意力机制和GAN的蛋白质二级结构预测[J].中国科技论文在线精品论文,2023,16(02):148-159.
LI S, YUAN L, MA Y M, et al. WG-ICRN: Protein 8-state secondary structure prediction based on Wasserstein generative adversarial networks and residual networks with Inception modules [J]. Mathematical Biosciences and Engineering, 2023, 20(5): 7721-37.
MADDHURI VENKATA SUBRAMANIYA S R, TERASHI G, JAIN A, et al. Protein Contact Map Denoising Using Generative Adversarial Networks [J]. bioRxiv, 2020.
Fang, F. A. N. G., Tan, W., & Liu, J. Z. (2005). Tuning of coordinated controllers for boiler-turbine units. Acta Automatica Sinica, 31(2), 291-296.
Lv, Y., Fang, F. A. N. G., Yang, T., & Romero, C. E. (2020). An early fault detection method for induced draft fans based on MSET with informative memory matrix selection. ISA transactions, 102, 325-334.
Zhang, X., Fang, F., & Liu, J. (2019). Weather-classification-MARS-based photovoltaic power forecasting for energy imbalance market. IEEE Transactions on Industrial Electronics, 66(11), 8692-8702.
Wei, L., & Fang, F. (2016). ${H} _ {infty} $-LQR-Based Coordinated Control for Large Coal-Fired Boiler–Turbine Generation Units. IEEE Transactions on Industrial Electronics, 64(6), 5212-5221.
Liu, J., Song, D., Li, Q., Yang, J., Hu, Y., Fang, F., & Joo, Y. H. (2023). Life cycle cost modelling and economic analysis of wind power: A state of art review. Energy Conversion and Management, 277, 116628.
Fang, F., Zhu, Z., Jin, S., & Hu, S. (2020). Two-layer game theoretic microgrid capacity optimization considering uncertainty of renewable energy. IEEE Systems Journal, 15(3), 4260-4271.
Fang, F., & Xiong, Y. (2014). Event-driven-based water level control for nuclear steam generators. IEEE Transactions on Industrial electronics, 61(10), 5480-5489.
Liu, J., Zeng, D., Tian, L., Gao, M., Wang, W., Niu, Y., & Fang, F. (2015). Control strategy for operating flexibility of coal-fired power plants in alternate electrical power systems. Proceedings of the CSEE, 35(21), 5385-5394.
Fang, F., & Wu, X. (2020). A win–win mode: The complementary and coexistence of 5G networks and edge computing. IEEE Internet of Things Journal, 8(6), 3983-4003.
Wang, N., Fang, F., & Feng, M. (2014, May). Multi-objective optimal analysis of comfort and energy management for intelligent buildings. In The 26th Chinese control and decision conference (2014 CCDC) (pp. 2783-2788). IEEE.
Wang, W., Liu, J., Zeng, D., Fang, F., & Niu, Y. (2020). Modeling and flexible load control of combined heat and power units. Applied Thermal Engineering, 166, 114624.
Lv, Y., Lv, X., Fang, F., Yang, T., & Romero, C. E. (2020). Adaptive selective catalytic reduction model development using typical operating data in coal-fired power plants. Energy, 192, 116589.
Fang, F., Jizhen, L., & Wen, T. (2004). Nonlinear internal model control for the boiler-turbine coordinate systems of power unit. PROCEEDINGS-CHINESE SOCIETY OF ELECTRICAL ENGINEERING, 24(4), 195-199.
Chang, K., Wang, Y., Ren, H., Wang, M., Liang, S., Han, Y., ... & Li, X. (2023). Chipgpt: How far are we from natural language hardware design. arXiv preprint arXiv:2305.14019.
Wang, Y., Han, Y., Zhang, L., Li, H., & Li, X. (2015, June). ProPRAM: Exploiting the transparent logic resources in non-volatile memory for near data computing. In Proceedings of the 52nd Annual Design Automation Conference (pp. 1-6).
Chen, W., Wang, Y., Yang, S., Liu, C., & Zhang, L. (2020, March). You only search once: A fast automation framework for single-stage dnn/accelerator co-design. In 2020 Design, Automation & Test in Europe Conference & Exhibition (DATE) (pp. 1283-1286). IEEE.
Hamdioui, S., Pouyan, P., Li, H., Wang, Y., Raychowdhur, A., & Yoon, I. (2017, November). Test and reliability of emerging non-volatile memories. In 2017 IEEE 26th Asian Test Symposium (ATS) (pp. 175-183). IEEE.
Ma, X., Wang, Y., Wang, Y., Cai, X., & Han, Y. (2022). Survey on chiplets: interface, interconnect and integration methodology. CCF Transactions on High Performance Computing, 4(1), 43-52.
Wu, B., Wang, C., Wang, Z., Wang, Y., Zhang, D., Liu, D., ... & Hu, X. S. (2020). Field-free 3T2SOT MRAM for non-volatile cache memories. IEEE Transactions on Circuits and Systems I: Regular Papers, 67(12), 4660-4669.
Zhao, X., Wang, Y., Liu, C., Shi, C., Tu, K., & Zhang, L. (2020, July). BitPruner: Network pruning for bit-serial accelerators. In 2020 57th ACM/IEEE Design Automation Conference (DAC) (pp. 1-6). IEEE.
Han, Y., Wang, Y., Li, H., & Li, X. (2014, November). Data-aware DRAM refresh to squeeze the margin of retention time in hybrid memory cube. In 2014 IEEE/ACM International Conference on Computer-Aided Design (ICCAD) (pp. 295-300). IEEE.
Wang, Y., Li, H., & Li, X. (2017). A case of on-chip memory subsystem design for low-power CNN accelerators. IEEE Transactions on Computer-Aided Design of Integrated Circuits and Systems, 37(10), 1971-1984.
Liu, C., Chu, C., Xu, D., Wang, Y., Wang, Q., Li, H., ... & Cheng, K. T. (2021). HyCA: A hybrid computing architecture for fault-tolerant deep learning. IEEE Transactions on Computer-Aided Design of Integrated Circuits and Systems, 41(10), 3400-3413.
Xu, D., Chu, C., Wang, Q., Liu, C., Wang, Y., Zhang, L., ... & Cheng, K. T. (2020, October). A hybrid computing architecture for fault-tolerant deep learning accelerators. In 2020 IEEE 38th International Conference on Computer Design (ICCD) (pp. 478-485). IEEE.
Wang, C., Wang, Y., Han, Y., Song, L., Quan, Z., Li, J., & Li, X. (2017, January). CNN-based object detection solutions for embedded heterogeneous multicore SoCs. In 2017 22nd Asia and South Pacific design automation conference (ASP-DAC) (pp. 105-110). IEEE.
Liu, B., Chen, X., Wang, Y., Han, Y., Li, J., Xu, H., & Li, X. (2019, January). Addressing the issue of processing element under-utilization in general-purpose systolic deep learning accelerators. In Proceedings of the 24th Asia and South Pacific Design Automation Conference (pp. 733-738).
Li, C., Wang, Y., Liu, C., Liang, S., Li, H., & Li, X. (2021). {GLIST}: Towards {in-storage} graph learning. In 2021 USENIX Annual Technical Conference (USENIX ATC 21) (pp. 225-238).
Qu, S., Li, B., Wang, Y., Xu, D., Zhao, X., & Zhang, L. (2020, July). RaQu: An automatic high-utilization CNN quantization and mapping framework for general-purpose RRAM accelerator. In 2020 57th ACM/IEEE Design Automation Conference (DAC) (pp. 1-6). IEEE.
Wang, Y., Deng, J., Fang, Y., Li, H., & Li, X. (2017). Resilience-aware frequency tuning for neural-network-based approximate computing chips. IEEE Transactions on Very Large Scale Integration (VLSI) Systems, 25(10), 2736-2748.
Li, W., Wang, Y., Li, H., & Li, X. (2019, January). P3M: a PIM-based neural network model protection scheme for deep learning accelerator. In Proceedings of the 24th Asia and South Pacific Design Automation Conference (pp. 633-638).
Xu, D., Zhu, Z., Liu, C., Wang, Y., Zhao, S., Zhang, L., ... & Cheng, K. T. (2021). Reliability evaluation and analysis of FPGA-based neural network acceleration system. IEEE Transactions on Very Large Scale Integration (VLSI) Systems, 29(3), 472-484.
Li, J., Chen, Z., Cheng, L., & Liu, X. (2022). Energy data generation with wasserstein deep convolutional generative adversarial networks. Energy, 257, 124694.
Liu, Q., Cheng, L., Alves, R., Ozcelebi, T., Kuipers, F., Xu, G., ... & Chen, S. (2021). Cluster-based flow control in hybrid software-defined wireless sensor networks. Computer Networks, 187, 107788.
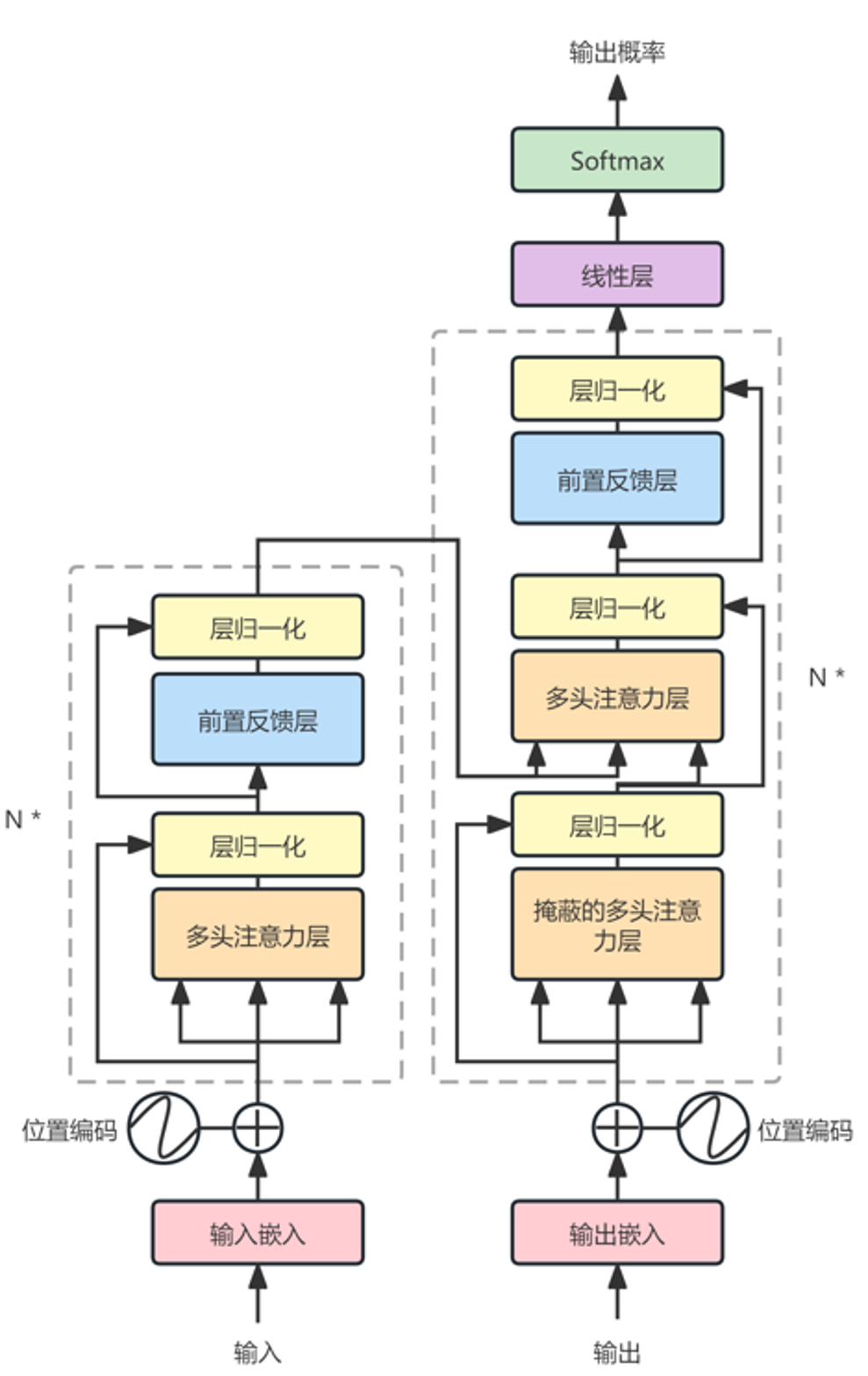