基于拮抗特性模型的夜视微光图像与红外图像彩色融合
DOI:
https://doi.org/10.52810/FAAI.2024.004关键词:
计算机视觉, 特征跟踪, 光流法, 视觉特征, 视觉跟踪摘要
融合可以有效地利用可见光图像的色彩信息得到较好的可视效果,又可以充分利用红外图像获得人眼无法观察到的红外信息,具有广阔的应用前景。论文首先采用拮抗特性模型中的中心-周边对抗网络对红外与微光图像进行增强,利用区域生长方法对增强后红外图像进行分割,根据分割后各区域亮度得到目标图像。在融合阶段加入两种融合运算:采用选择运算将目标图像信息融合到增强后微光背景中作为亮度通道的输入;利用拮抗特性模型将经采用区域生长法得到红外图像和增强后的微光图像进行融合,作为饱和度通道的输入。同时,直接将增强的微光图像送入调色通道, 作为彩色融合图像的背景进行调色,最后通过彩色重映射并加以显示。实验结果获得的图像具有较好的目标指示特性,色彩更适合人眼观察,有利于提高对目标情景的感知能力。
参考文献
蒋晓瑜, 裴闯, 汪熙, 谢之宇. (2010). 一种面向目标的伪彩色图像融合算法. 装甲兵工程学院学报, 24(6), 55-58.
张纪乾. (2024). 热红外与可见光图像融合方法研究[D]. 西安理工大学.
沈英, 黄春红, 黄峰, 等. (2021). 红外与可见光图像融合技术的研究进展[J]. 红外与激光工程, 50(09), 152-169.
高文, 朱明, 郝志成. (2016). 彩色夜视技术的研究进展[J]. 液晶与显示, 31(12), 1168-1179.
Zhou, H. (2012). An stationary wavelet transform and curvelet transform based infrared and visible images fusion algorithm. International Journal of Digital Content Technology and its Applications, 6(1), 144-151.
Liu, Y., Qi, Z., Cheng, J., & Chen, X. (2024). Rethinking the Effectiveness of Objective Evaluation Metrics in Multi-focus Image Fusion: A Statistic-based Approach. IEEE Transactions on Pattern Analysis and Machine Intelligence. doi: 10.1109/TPAMI.2024.3367905.
He, W., Guo, Y., & Gao, C. (2011). An adaptive color fusion method for infrared and visible images. ICIC Express Letters, 5(7), 2359-2364.
李立柱, 孟明, 高云园, 等. (2023). 基于小波变换的EEG-fNIRS多模态数据融合方法[J]. 传感技术学报, 36(07), 1064-1072.
Shao, Z., Liu, J., & Cheng, Q. (2012). Fusion of infrared and visible images based on focus measure operators in the curvelet domain. Applied Optics, 51(12), 1910-1921.
Adu, J., Wang, M., Wu, Z., & Hu, J. (2012). Infrared image and visible light image fusion based on nonsubsampled contourlet transform and the gradient of uniformity. International Journal of Advancements in Computing Technology, 4(5), 114-121.
朱进. (2021). 微光/热红外图像色彩传递及多尺度分解融合算法研究[D]. 北京理工大学.
张阵. (2019). 红外与微光图像彩色融合仿生算法的研究与实现[D]. 武汉理工大学.
周丁. (2023). 红外图像与可见光图像的融合研究[D]. 江苏科技大学.
林怡彤. (2024). 基于图像增强与对抗网络的夜视图像彩色化[D]. 湖南大学.
van Kerkoerle, T., Self, M., & Roelfsema, P. (2017). Layer-specificity in the effects of attention and working memory on activity in primary visual cortex. Nat Commun, 8, 13804.
Fang, F. A. N. G., Tan, W., & Liu, J. Z. (2005). Tuning of coordinated controllers for boiler-turbine units. Acta Automatica Sinica, 31(2), 291-296.
Wei, L., & Fang, F. (2016). ${H} _ {infty} $-LQR-Based Coordinated Control for Large Coal-Fired Boiler–Turbine Generation Units. IEEE Transactions on Industrial Electronics, 64(6), 5212-5221.
Wang, W., Liu, J., Zeng, D., Fang, F., & Niu, Y. (2020). Modeling and flexible load control of combined heat and power units. Applied Thermal Engineering, 166, 114624.
Liu, J., Song, D., Li, Q., Yang, J., Hu, Y., Fang, F., & Joo, Y. H. (2023). Life cycle cost modelling and economic analysis of wind power: A state of art review. Energy Conversion and Management, 277, 116628.
Fang, F., Zhu, Z., Jin, S., & Hu, S. (2020). Two-layer game theoretic microgrid capacity optimization considering uncertainty of renewable energy. IEEE Systems Journal, 15(3), 4260-4271.
Liu, J., Zeng, D., Tian, L., Gao, M., Wang, W., Niu, Y., & Fang, F. (2015). Control strategy for operating flexibility of coal-fired power plants in alternate electrical power systems. Proceedings of the CSEE, 35(21), 5385-5394.
Fang, F., & Xiong, Y. (2014). Event-driven-based water level control for nuclear steam generators. IEEE Transactions on Industrial electronics, 61(10), 5480-5489.
Lv, Y., Lv, X., Fang, F., Yang, T., & Romero, C. E. (2020). Adaptive selective catalytic reduction model development using typical operating data in coal-fired power plants. Energy, 192, 116589.
Fang, F., & Wu, X. (2020). A win–win mode: The complementary and coexistence of 5G networks and edge computing. IEEE Internet of Things Journal, 8(6), 3983-4003.
Lv, Y., Fang, F. A. N. G., Yang, T., & Romero, C. E. (2020). An early fault detection method for induced draft fans based on MSET with informative memory matrix selection. ISA transactions, 102, 325-334.
Wang, N., Fang, F., & Feng, M. (2014, May). Multi-objective optimal analysis of comfort and energy management for intelligent buildings. In The 26th Chinese control and decision conference (2014 CCDC) (pp. 2783-2788). IEEE.
Fang, F., Jizhen, L., & Wen, T. (2004). Nonlinear internal model control for the boiler-turbine coordinate systems of power unit. PROCEEDINGS-CHINESE SOCIETY OF ELECTRICAL ENGINEERING, 24(4), 195-199.
Li, W., Wang, Y., Li, H., & Li, X. (2019, January). P3M: a PIM-based neural network model protection scheme for deep learning accelerator. In Proceedings of the 24th Asia and South Pacific Design Automation Conference (pp. 633-638).
Wang, Y., Deng, J., Fang, Y., Li, H., & Li, X. (2017). Resilience-aware frequency tuning for neural-network-based approximate computing chips. IEEE Transactions on Very Large Scale Integration (VLSI) Systems, 25(10), 2736-2748.
Qu, S., Li, B., Wang, Y., Xu, D., Zhao, X., & Zhang, L. (2020, July). RaQu: An automatic high-utilization CNN quantization and mapping framework for general-purpose RRAM accelerator. In 2020 57th ACM/IEEE Design Automation Conference (DAC) (pp. 1-6). IEEE.
Li, C., Wang, Y., Liu, C., Liang, S., Li, H., & Li, X. (2021). {GLIST}: Towards {in-storage} graph learning. In 2021 USENIX Annual Technical Conference (USENIX ATC 21) (pp. 225-238).
Liu, B., Chen, X., Wang, Y., Han, Y., Li, J., Xu, H., & Li, X. (2019, January). Addressing the issue of processing element under-utilization in general-purpose systolic deep learning accelerators. In Proceedings of the 24th Asia and South Pacific Design Automation Conference (pp. 733-738).
Wang, C., Wang, Y., Han, Y., Song, L., Quan, Z., Li, J., & Li, X. (2017, January). CNN-based object detection solutions for embedded heterogeneous multicore SoCs. In 2017 22nd Asia and South Pacific design automation conference (ASP-DAC) (pp. 105-110). IEEE.
Xu, D., Chu, C., Wang, Q., Liu, C., Wang, Y., Zhang, L., ... & Cheng, K. T. (2020, October). A hybrid computing architecture for fault-tolerant deep learning accelerators. In 2020 IEEE 38th International Conference on Computer Design (ICCD) (pp. 478-485). IEEE.
Liu, C., Chu, C., Xu, D., Wang, Y., Wang, Q., Li, H., ... & Cheng, K. T. (2021). HyCA: A hybrid computing architecture for fault-tolerant deep learning. IEEE Transactions on Computer-Aided Design of Integrated Circuits and Systems, 41(10), 3400-3413.
Chen, W., Wang, Y., Yang, S., Liu, C., & Zhang, L. (2020, March). You only search once: A fast automation framework for single-stage dnn/accelerator co-design. In 2020 Design, Automation & Test in Europe Conference & Exhibition (DATE) (pp. 1283-1286). IEEE.
Wang, Y., Li, H., & Li, X. (2017). A case of on-chip memory subsystem design for low-power CNN accelerators. IEEE Transactions on Computer-Aided Design of Integrated Circuits and Systems, 37(10), 1971-1984.
Liu, Q., Cheng, L., Ozcelebi, T., Murphy, J., & Lukkien, J. (2019, May). Deep reinforcement learning for IoT network dynamic clustering in edge computing. In 2019 19th IEEE/ACM international symposium on cluster, Cloud and Grid Computing (CCGRID) (pp. 600-603). IEEE.
Hamdioui, S., Pouyan, P., Li, H., Wang, Y., Raychowdhur, A., & Yoon, I. (2017, November). Test and reliability of emerging non-volatile memories. In 2017 IEEE 26th Asian Test Symposium (ATS) (pp. 175-183). IEEE.
Ma, X., Wang, Y., Wang, Y., Cai, X., & Han, Y. (2022). Survey on chiplets: interface, interconnect and integration methodology. CCF Transactions on High Performance Computing, 4(1), 43-52.
Wu, B., Wang, C., Wang, Z., Wang, Y., Zhang, D., Liu, D., ... & Hu, X. S. (2020). Field-free 3T2SOT MRAM for non-volatile cache memories. IEEE Transactions on Circuits and Systems I: Regular Papers, 67(12), 4660-4669.
Zhao, X., Wang, Y., Liu, C., Shi, C., Tu, K., & Zhang, L. (2020, July). BitPruner: Network pruning for bit-serial accelerators. In 2020 57th ACM/IEEE Design Automation Conference (DAC) (pp. 1-6). IEEE.
Han, Y., Wang, Y., Li, H., & Li, X. (2014, November). Data-aware DRAM refresh to squeeze the margin of retention time in hybrid memory cube. In 2014 IEEE/ACM International Conference on Computer-Aided Design (ICCAD) (pp. 295-300). IEEE.
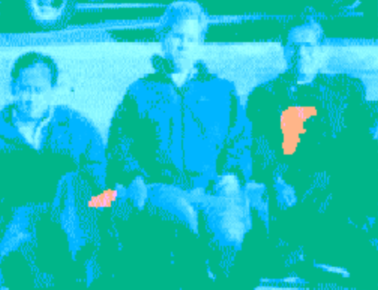